The Essential Role of Data Labeling in Modern Business
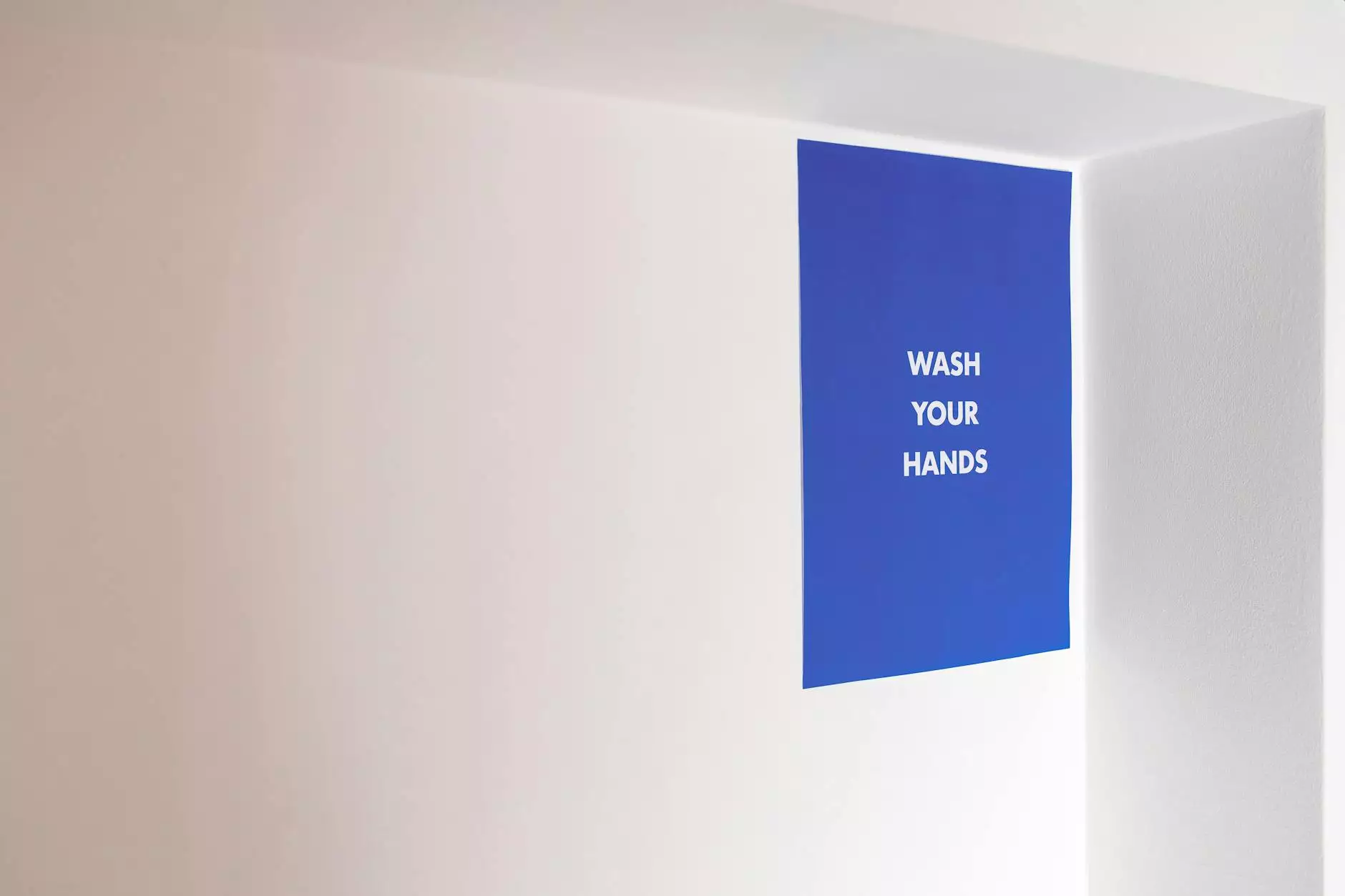
Data labeling has become a critical component for businesses leveraging artificial intelligence (AI) and machine learning (ML). As organizations strive to harness the potential of data, accurately labeling that data is paramount. This article explores the various facets of data labeling, its significance in today's digital landscape, and how it enhances business operations, boosts productivity, and drives decision-making.
Understanding Data Labeling
At its core, data labeling is the process of annotating or tagging data so that machines can understand it. This includes categorizing images, transcribing audio, annotating text, and much more. By adding relevant labels, businesses can train AI models to recognize patterns, make predictions, and automate tasks efficiently.
The Importance of Data Labeling in AI and ML
In the realm of AI and ML, the quality of data is crucial for successful outcomes. High-quality, accurately labeled data enables models to learn and generalize better. Here are some reasons why data labeling is indispensable:
- Enhanced Model Performance: Well-labeled data leads to improved accuracy in AI models, which results in better predictions and insights.
- Reduction in Training Time: Accurate labeling speeds up the training process, reducing the time AI models take to learn effectively.
- Informed Decision Making: Enterprises can make strategic decisions backed by data insights derived from well-labeled datasets.
- Competitive Advantage: Businesses that leverage properly labeled data can outperform competitors who fail to do so.
Types of Data Labeling
Data labeling encompasses a variety of techniques, each serving distinct purposes based on the business requirements:
1. Image Annotation
Image annotation is a typical example of data labeling. It involves identifying objects within images and tagging them. This is essential for applications such as:
- Autonomous vehicles that need to recognize pedestrians, traffic signs, and other vehicles.
- Facial recognition systems that require labeled faces in images.
2. Text Annotation
This involves labeling text data to enable sentiment analysis, spam detection, or categorization. Companies use text annotation for:
- Natural Language Processing (NLP) applications.
- Chatbot development.
3. Video Annotation
Video data is annotated frame-by-frame for various applications, such as:
- Surveillance systems using behavior analysis.
- Sports analytics that track player movements.
Data Annotation Tools and Platforms
For businesses looking to enhance their data labeling processes, utilizing a dedicated data annotation tool or platform is essential. These platforms offer various features that streamline the labeling process, improve accuracy, and save time. Here are some notable features to look for:
- User-Friendly Interface: A simple, intuitive design that allows teams to annotate data quickly and efficiently.
- Collaboration Features: Tools that facilitate teamwork and streamline communication among annotators.
- Quality Control Mechanisms: Processes that ensure the accuracy and consistency of labels.
- Integration Capabilities: Ability to integrate with other tools and platforms used in the data pipeline.
How Data Labeling Influences Business Growth
Investing in data labeling significantly impacts business growth. Here’s how:
Improved Customer Insights
With accurate data labeling, businesses can analyze customer behavior and preferences. This allows for tailored marketing strategies, improved product offerings, and enhanced customer satisfaction.
Increased Operational Efficiency
Automating processes with AI systems trained on labeled data reduces manual workload. Businesses can streamline operations, minimize errors, and allocate resources more effectively.
Fostering Innovation
With rich, well-labeled datasets, organizations can explore new applications for AI and ML, fostering innovation and keeping pace with industry trends.
Challenges in Data Labeling
Despite its benefits, data labeling comes with challenges:
1. Time-Consuming Process
Labeling large datasets can be time-consuming, which can delay project timelines. Efficient tools and well-defined processes can help mitigate this.
2. Human Error
When data labeling is performed manually, there is a risk of human error. Implementing quality control measures is vital to avoid inaccuracies.
3. Scalability Issues
As the size of data grows, scaling the labeling operations can be challenging. Businesses need to develop strategies or invest in scalable solutions.
Best Practices for Effective Data Labeling
To maximize the benefits of data labeling, businesses should consider the following best practices:
- Define Clear Guidelines: Create detailed annotation guidelines to ensure consistency across the team.
- Utilize a Combination of Automated and Manual Labeling: Use automation for initial labeling and manual verification for accuracy.
- Implement Iterative Feedback Loops: Continuously improve the labeling process by seeking feedback from annotators and stakeholders.
- Invest in Training: Provide regular training to annotators to enhance their understanding of the subject matter and labeling techniques.
Conclusion
As businesses continue to harness the power of data in their operations, the significance of data labeling cannot be overstated. By investing in high-quality labeling processes, utilizing effective data annotation tools and platforms, and adhering to best practices, organizations can drive meaningful results, gain valuable insights, and ultimately achieve sustainable growth. As the landscape of AI and data continues to evolve, staying ahead with robust labeling strategies is essential for success in today's competitive business environment.
Visit keylabs.ai to learn more about how we can help you with data annotation tools and platforms tailored to your business needs.