Labeling Training Data: Transforming Keymakr's Home Services with Precision
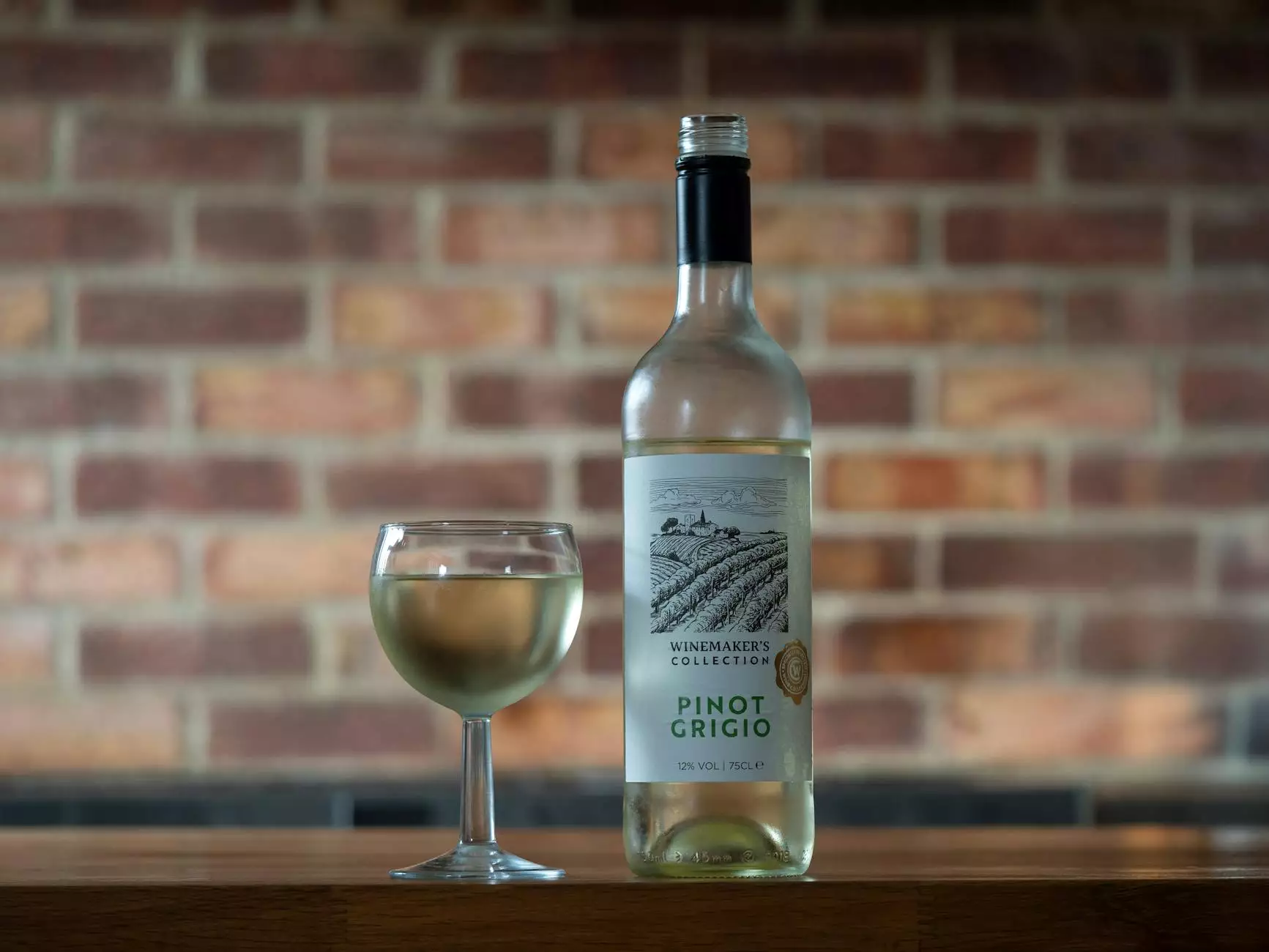
Data is the new oil, and its refinement is essential for organizations to thrive in today’s competitive landscape. For businesses like Keymakr, which specializes in Home Services, Keys & Locksmiths, leveraging the power of labeling training data becomes critical in enhancing service delivery, efficiency, and customer satisfaction.
Understanding Labeling Training Data
To appreciate the impact of labeling training data, it’s crucial to comprehend what it entails. Essentially, labeling training data involves annotating raw data with tags that provide context, allowing machine learning models to learn from them effectively. This process transforms unstructured data into structured datasets that machines can understand and process.
Why is Labeling Training Data Important?
Labeling training data is paramount for various reasons, especially when considering its application within Keymakr:
- Enhanced Predictions: Accurate labeling results in improved predictions by models, which can streamline service requests based on customer needs.
- Optimized Resource Allocation: By understanding service patterns, Keymakr can allocate resources more efficiently, responding to customer needs promptly.
- Improved Customer Experience: Faster and more accurate services lead to higher customer satisfaction rates, which are crucial in a service-oriented business.
- Cost Efficiency: Reducing the time and effort required to process requests results in significant cost savings for operational processes.
The Role of Machine Learning in Keymakr’s Business
Incorporating machine learning into Keymakr’s operations requires a well-defined strategy for handling labeling training data. The integration of cutting-edge technologies can elevate the standard of services offered by locksmiths and home service professionals.
Applications of Machine Learning in Home Services
Here’s how machine learning reshapes Keymakr’s home service offerings through data labeling:
- Predictive Maintenance: By predicting when a lock may need maintenance, Keymakr minimizes unexpected breakdowns.
- Customer Segmentation: Analyzing customer data helps in tailoring services to meet specific demographic needs.
- Anomaly Detection: Machine learning can spot breaches of typical usage patterns, enhancing security alerts for clients.
- Automating Responses: Training data can enable automated systems to respond to common customer inquiries quickly.
The Process of Labeling Training Data at Keymakr
To successfully harness the benefits of data labeling, Keymakr implements a structured approach. Here are the primary steps involved in labeling training data:
1. Data Collection
The first step in the process involves collecting raw data from various sources, such as:
- Customer inquiry logs
- Service execution reports
- Feedback and reviews
2. Data Annotation
Following the collection phase, the data is annotated. This involves tagging data points with relevant labels that provide context for machine learning models. For instance:
- Feedback Classification: Annotating reviews as positive, negative, or neutral helps train sentiment analysis models.
- Service Type Identification: Labeling service requests according to type (e.g., lock repair, installation) aids in predictive maintenance models.
3. Model Training
Once labeled, the data is fed into machine learning algorithms. The models are trained to recognize patterns and make predictions based on newly acquired knowledge. This is crucial for enhancing Keymakr’s automated services.
4. Testing and Validation
After training, models undergo rigorous testing to ensure their predictions align with real-world outcomes. Cross-validation with withheld datasets ensures the model’s accuracy and reliability.
Case Study: Keymakr's Success with Labeling Training Data
To illustrate the significance of labeling training data, consider the following case study involving Keymakr's locksmith services:
The Challenge
Keymakr faced challenges in meeting varying customer demands during peak times while ensuring prompt service delivery. This inconsistency impacted customer satisfaction and service quality.
Implementing a Solution
By effectively labeling training data relating to customer inquiries and service completion logs, Keymakr implemented machine learning solutions to predict service demands. Key indicators such as seasonal trends and common request types were prioritized through data analysis.
The Outcome
The results were astounding:
- 30% Reduction in Response Time: Improved prediction models allowed for quicker allocation of resources based on anticipated demand.
- 20% Increased Customer Satisfaction: Faster service delivery led to happier clients, as shown through enhanced feedback ratings.
- 15% Cost Savings: Efficient operations allowed Keymakr to save on overtime costs by deploying staff strategically.
Challenges in Labeling Training Data
While labeling training data delivers numerous benefits, organizations like Keymakr may encounter challenges during the process:
- Data Privacy Concerns: Ensuring that customer data is managed appropriately and ethically, complying with regulations such as GDPR, is paramount.
- Resource Intensive: The labeling process can be time-consuming and often requires skilled personnel to ensure quality annotations.
- Quality Control: Maintaining high standards in data labeling and ensuring the accuracy of labeled data can be challenging.
The Future of Labeling Training Data and Keymakr
As technology continues to evolve, the future of labeling training data looks promising for Keymakr. Advancements in artificial intelligence (AI) and automation are poised to streamline the data labeling process further. Here are potential future trends:
1. Enhanced Automation
With the development of sophisticated AI, automation in labeling could potentially reduce human intervention, allowing models to learn from vast datasets without extensive manual input.
2. Real-Time Data Processing
Future advancements may allow Keymakr to process labeling data in real time, leading to instantaneous service enhancements based on live customer feedback.
3. Predictive Analytics
As Keymakr continues refining its use of both labeled and unlabeled data, predictive analytics will shape proactive service delivery, anticipating customer needs ahead of time.
Conclusion
The revolutionary impact of labeling training data cannot be overstated. For Keymakr, the implementation of structured training data approaches not only drives efficiency but fosters an unparalleled customer experience in home services and locksmith operations. As technology continues to advance, Keymakr's commitment to leveraging data-driven insights reinforces its position as a leader in an increasingly competitive market. Embracing these innovations today will build a stronger, customer-focused tomorrow.