Unlocking the Power of Semantic Segmentation Video in Data Annotation
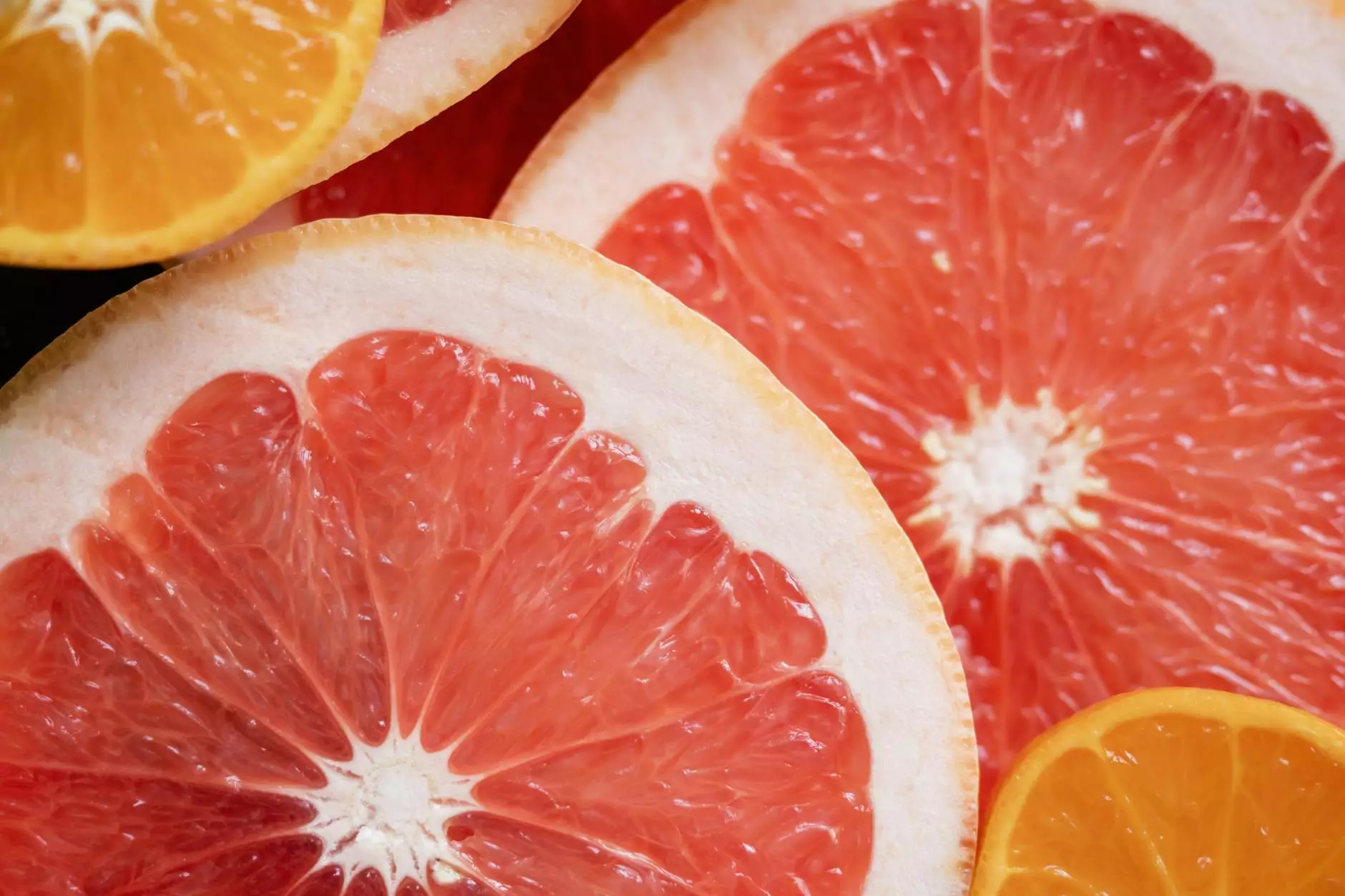
In the age of artificial intelligence and machine learning, the demand for accurate data annotation has surged dramatically. One of the most effective techniques employed in this domain is semantic segmentation video, which plays a pivotal role in training algorithms for better understanding and processing of visual data.
Understanding Semantic Segmentation Video
Semantic segmentation is a computer vision task that involves partitioning an image into regions and classifying each region into relevant classes. When the same technique is applied to video, it introduces a dynamic element, as the model learns not only from static images but also from the context of how objects interact and move over time.
The Role of Data Annotation in Semantic Segmentation
At the core of semantic segmentation is the process of data annotation. Effective data annotation is crucial for any AI model's success, especially in fields such as autonomous driving, augmented reality, and robotics. Here's why:
- Accuracy: Precise annotations ensure that the AI can correctly identify and classify objects in different environments.
- Contextual Understanding: Video data provides context, which enhances the AI’s ability to recognize and predict actions or interactions.
- Scalability: With automated tools like those provided by Keylabs.ai, businesses can scale their data annotation processes efficiently.
The Benefits of Semantic Segmentation Video
Implementing semantic segmentation in video annotation brings numerous advantages to businesses, particularly in the technology landscape:
1. Enhanced Object Recognition
Semantic segmentation video facilitates improved object detection by classifying pixels in videos, allowing for accurate differentiation between various objects like people, vehicles, and other entities. This granularity in data helps models better understand their operational environment and enhances performance significantly.
2. Improved Model Training
Using well-annotated video data allows machine learning models to train on diverse and high-quality datasets, leading to higher validation accuracy and robustness in real-world applications. Properly annotated video data enhances the learning process by providing essential context for temporal data.
3. Real-Time Applications
In industries such as security and surveillance, semantic segmentation video assists in real-time monitoring and analysis, identifying suspicious activities or tracking movements efficiently. This capability is crucial for developing responsive systems that require immediate decision-making based on visual cues.
4. Cost-Efficiency
By utilizing platforms that specialize in data annotation like Keylabs.ai, businesses can reduce operational costs associated with manual data processing while achieving high-quality annotations suited for semantic segmentation needs.
How Keylabs.ai Innovates Data Annotation Processes
Keylabs.ai stands out in the realm of data annotation tools and platforms, particularly with its emphasis on advanced technologies like semantic segmentation video annotation. Here’s how:
1. Advanced Technologies
Keylabs.ai integrates state-of-the-art technologies to automate the annotation process, ensuring that businesses can annotate their video data rapidly and accurately. The innovative systems manage vast datasets, enhancing productivity and ensuring consistency across annotations.
2. User-Friendly Interface
The platform’s interface is designed with end-users in mind, making it accessible to individuals regardless of their technical expertise. This democratization of technology empowers teams to collaborate effectively on data annotation projects without significant barriers to entry.
3. Comprehensive Support
Keylabs.ai provides comprehensive support and training to clients, ensuring that businesses can maximize the utility of their data annotation tools and platforms. Their customer service is tailored to assist enterprises in overcoming challenges related to data annotation.
4. Customizable Solutions
Every business has unique requirements, and Keylabs.ai understands that. The platform offers customizable annotation solutions that cater to specific needs, allowing businesses to optimize their annotation workflows for different types of video data.
Applications of Semantic Segmentation Video Across Industries
The influence of semantic segmentation video isn’t limited to one sector; its applications extend across various industries:
1. Automotive and Transportation
In the automotive industry, semantic segmentation is pivotal for developing autonomous driving systems. Accurate segmentation of roadway elements such as lanes, vehicles, and pedestrians is essential to ensure safety and operational efficiency.
2. Healthcare
In healthcare, video analysis through semantic segmentation aids in monitoring patient activities and detecting anomalies during surgical procedures or regular health checks. This application enhances patient safety and care delivery.
3. Retail and E-commerce
Retailers leverage video segmentation to analyze customer behavior, track foot traffic, and optimize store layouts. Through understanding customer movements, businesses can enhance the shopping experience substantially.
4. Entertainment and Multimedia
In the entertainment sector, semantic segmentation video empowers content creators to enrich visual storytelling by applying effects or filters that depend on real-time analysis of the video content.
The Future of Semantic Segmentation in Video
As technology advances, the future of semantic segmentation video looks promising. Innovations in machine learning and computer vision will likely lead to more sophisticated approaches in video analysis, paving the way for enhanced applications that will transform industries.
1. Increased Automation
With the rise of automated data annotation tools, businesses will increasingly rely on these solutions for more efficient data processing, reducing time and costs associated with manual annotations.
2. Real-Time Processing Capabilities
Further developments in real-time video processing and AI capabilities will allow for instantaneous video annotation, enabling applications that require immediate feedback and action.
3. Enhanced Learning Algorithms
Future algorithms will become more advanced and capable of learning from fewer annotated examples, thus making semantic segmentation video more powerful and accessible to various business needs.
Conclusion
Semantic segmentation video is a crucial technique for businesses looking to leverage visual data for artificial intelligence applications. By adopting advanced data annotation tools provided by platforms like Keylabs.ai, enterprises can enhance their data processes, improve model performances, and ultimately gain a competitive edge in today’s data-driven landscape.
In an era defined by rapid technological advancements, investing in effective data annotation through semantic segmentation video will enable businesses not only to keep pace with industry demands but also to innovate and thrive.